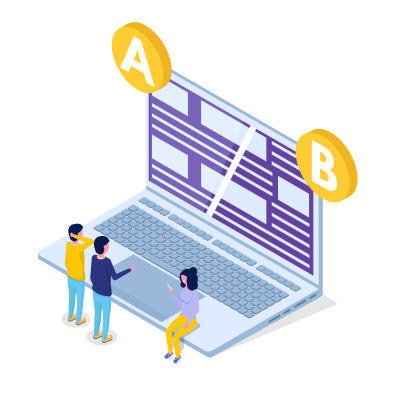
Adaptable Splits
Automatically learn the optimal traffic split to maximize revenue while keeping an eye for new opportunities.
Brands like Netflix, Booking, Hubspot and Expedia have all discovered the potential in Contextual Bandits for growing their business.
Bandit algorithms have shown 60% reduction in cost when compared to traditional A/B testing.
Automatically learn the optimal traffic split to maximize revenue while keeping an eye for new opportunities.
Contextual bandits take into account user features (e.g. geography, time of day, history) and learn the best match between user and content.
The process is completely automated, no need to babysit (AB-sit) until convergence.
We specialize in implementing bandit algorithms in production using state-of-the-art Machine learning and AI.
We have extensive experience customizing bandit algorithms to your specific objective.
We help you converge better on the optimal variant and reduce advertising budget significantly.
We help you dynamically target your audience using advanced machine learning and feature engineering.
We've gathered use-cases from our clients and we'll demonstrate the effect bandits had on their bottom-line.
We've received a lot of interest in recent years, here are some of the most common questions.
Contextual Bandits require a more advanced tech stack than A/B testing, we recommend clients to start their journey by exploring the potential on traditional A/B testing first.
MV-testing, is essentially a split of more than 2 variants (A-B-C-D testing). They do not account for the similarity between variants (e.g. both A and C have the same background) and therefore the convergence is slower.
Recommendation is tricky. On the one hand, you'd like to suggest the items that sell well. On the other hand, you don't want to miss new opportunities and trends. Bandits are a great trade-off between exploration and exploitation.
Assume you have a product page, and you need to control the position of elements, the key figure and the backgound. Contextual Bandits test and find the best combination for your audience dynamically.
Latest news, tips and best practices.
In this episode we introduce the basic concept of A/B testing and the assumption it makes. We discuss the monitoring phase (aka "AB-sitting") and the role of the account manager.
We all want quick results, but stopping an experiment too quickly can cause suboptimal results. In this episode we'll discuss statistical significance and stopping criteria.
Have more than 2 alternatives to test? Welcome to the realm of multivariate testing. Are some of them related to one another ? maybe you should consider a factorial design.
Drop us a line